Les notions, essentiellement philosophiques, de volonté et de liberté sont au cœur de la matière.
– Emmanuel Gaillard
Arbitration is a form of private dispute resolution. Parties arbitrate disputes through a private system created of their own will and liberty, contractually exiting the public, state-controlled system of dispute resolution. Whilst there are many different types of arbitration, most remain subject to similar challenges, such as the constitution of the tribunal, the seat of the arbitration, and the applicable law. Any law student need only refer to Lord Justice Kerr’s 1987 parable, the Macao Sardine Case, to grasp many of the basic concepts in arbitration.1)Kerr, L. J. (1987). “Arbitration v. Litigation: The Macao Sardine Case.” Arbitration International 3(1): 79-86.
Artificial intelligence, according to the Oxford English Dictionary, is the capacity of computers or other machines to exhibit or simulate intelligent behaviour, or the field of study concerned with this, abbreviated AI. In the UK, the government has defined AI as technologies with the ability to perform tasks that would otherwise require human intelligence, such as visual perception, speech recognition, and language translation.
At first sight, arbitration, a system of private dispute resolution, and artificial intelligence, the ability of computers to exhibit intelligence or perform tasks, might appear to be strange bedfellows. But with AI permeating all aspects of life, questions for the law – and for arbitration – have been raised: how is AI being used? What is the potential for AI’s application in arbitration – and its risks? To begin answering these questions, one must first tackle a more fundamental one: what is AI, exactly?
Artificial (specific) intelligence
Artificial intelligence resists definition. Alan Turing first proposed the concept of a modern computer in a 1936 paper, dealing with an esoteric question concerning incompleteness in mathematical logic.2)Turing, A. (1937). “On Computable Numbers, with an Application to the Entscheidungsproblem.” Proceedings of The London Mathematical Society 41: 230-265. In 1950, he would pose the question, “can machines think?”, proposing the imitation game as a test for intelligence, now commonly referred to as the “Turing test”. Researchers in the United States would pick up the concept only a year after his early passing: “artificial intelligence” as a term or concept dates to 1955, set out in a proposal for the 1956 Dartmouth Summer Research Project.3)McCarthy, J., M. Minsky, N. Rochester and C. Shannon (1955, August 31). “A Proposal for the Dartmouth Summer Research Project on Artificial Intelligence.“ As one attendee, Allen Newell, recalled the definition discussed at the Dartmouth Project in a classic paper:4)Newell, A. (1973). “You can’t play 20 questions with nature and win: projective comments on the papers of this symposium.” Visual Information Processing: 283–308.
“AI is the field devoted to building artifacts that are intelligent, where ‘intelligent’ is operationalized through intelligence tests…and other tests of mental ability.”
In reality, current AI systems are specific, narrowly-defined, and “dumb”. Much turns on identifying the test to be applied. In circumstances where a yet more basic question “what is intelligence?” has a multitude of answers, cross-pollination and debate continue between artificial intelligence and neuroscience.
Today, AI is most commonly a euphemism for automation, and dominated by a sub-branch of the field known as machine learning: computer systems that improve automatically through data and experience. Such “AI-driven” algorithmic systems are already here: from traffic control to voice-controlled digital assistants (such as Apple’s Siri and Google’s Alexa). Or, as John McCarthy wryly remarked, “as soon as it works, no one calls it AI anymore.”
Established applications in arbitration
As AI systems require sufficiently large, consistent, and reliable datasets to operate effectively, their application is limited in the context of a form of private dispute resolution where confidentiality is often paramount. However, a few applications stand out.
First, technology-assisted review. It is unsurprising that the most established technological solution for arbitration (or any dispute resolution process) is technology-assisted review for document disclosure, given the quantity of underlying data available. The definition of “document” has embraced the entire range of modern electronically-stored information (and meta-information), from the humble plain text file to entire SQL databases, regardless of format. In litigation, the courts have in the past 10 years started to recognise the value of document triage by AI-driven review platforms: see Pyrrho Investments v MWB Property [2016] EWHC 256 (Ch) in England (3.1 million documents).5)See also Brown v BCA Trading Ltd [2016] EWHC 1464 (Ch), where the order was contested. In other jurisdictions: in Australia, McConnell Dowell Constructors (Aust) Pty Ltd v. Santam Ltd (No 1) [2016] VSC 734; Vickery J. at [18] to [31] (1.4 million documents); in the US, Da Silva Moore v Publicis Groupe 11 Civ.1279 (ALC)(AJP), 24 February 2012, Southern District of New York (3 million documents); in Ireland, Irish Bank Resolution Corporation Ltd v Quinn [2015] IEHC 175; Fullam J. at [17] to [30] (1.7 million documents). Typically, a manual review is conducted on a training set representative of the universe of documents, with its output processed by the review platform; protocols are then implemented to ensure constant review and improvement. Such systems are, generally, types of supervised learning (a sub-category of machine learning). There is, however, a natural cap on efficiency: due to the largely heterogenous nature of arbitration, the process starts from scratch for each new case.
Second, AI-driven legal analysis. Two sub-groups can be distinguished here: legal analytics and predictive analysis.
Legal analytics has developed gradually, from basic data extraction and classification, traditionally associated with LexisNexis or Thomson Reuters Westlaw, to advanced analysis platforms such as ROSS Intelligence. These services benefit most legal practice areas, including arbitration. However, given the confidential nature of arbitration, penetration of legal analytics is shallow. For example, statistical analysis of cases and trends remain the eminent domain of arbitral institutions, who have privileged access to data. Third party offerings such as Jus Mundi have only started gaining ground comparatively recently.
In parallel, predictive analysis has been of interest to many: given enough data, AI can estimate the outcome of a case with high accuracy (or, at least do no worse than a legal expert).
One example is “Marshal”, an algorithm that predicts the outcome of cases before the Supreme Court of the United States, with 71% accuracy.6)Katz, D. M., M. J. Bommarito and J. Blackman (2017). “A general approach for predicting the behavior of the Supreme Court of the United States.” PLoS One 12(4). However, the volume of data required to achieve this prediction is not easily collected or structured, even in investor-state arbitrations where data may be more accessible, let alone private commercial arbitration. Marshal itself was programmed on 249,793 docket votes mapped against 1,501 engineered features, mined from the Supreme Court Database with judgments dating back to 1791.
In another study, researchers achieved prediction accuracy of around 90%; however, the underlying dataset required significant manual review and analysis.7)Shaikh, R. A., T. P. Sahu and V. Anand (2020). “Predicting Outcomes of Legal Cases based on Legal Factors using Classifiers.” Procedia Computer Science 167: 2393-2402.
That said, the British and Irish Legal Information Institute (BAILII) has recently granted access to its database of 400,000 searchable cases to the “AI and English Law” research team at the University of Oxford. Predictive analysis might remain prohibited by BAILII under this arrangement, but permitting natural language processing (NLP) is a concession. Watch this space.
The potential of AI
Will AI offer benefits for arbitration in the future?
It is conceivable that legal drafting could be automated, in light of advances in NLP, such as OpenAI’s Generative Pre-trained Transformer 3 (GPT-3) language model, released in 2020. GPT-3 is able to produce sensible text that is indistinguishable from any written text by a human, the first generation of any language model to achieve this feat. Even though there are clear and substantial limitations in its ability, the researchers cautioned the model’s potential dangers, including abuse of legal processes. They noted, however, that language models that produce high quality text generation might improve access to justice by lower existing barriers to carrying out activities that require human penmanship and increase efficacy.
Well-trained systems could also, in the future, communicate like humans. Google engineers recently trained an open-domain chatbot, Meena, to achieve 79% on a “Sensibleness and Specificity Average” test, within striking distance of human performance (86%), conversing in English and Chinese. However, the social conversation style assessed was relatively simple and deeper conversation remains untested (and difficult to test), with the researchers noting that achieving human-likeness is an incredibly broad and abstract concept. Whether an artificial machine can play the role of an arbitral participant is a tantalising prospect.
And can an AI system go further? Advances have been remarkable, often producing results years ahead of expectation. For example, Google DeepMind’s AlphaGo programme has defeated the best human players of Go (圍棋), generally considered to be the most complex traditional board game, and its AlphaFold programme has recently solved one of biology’s biggest challenges, correctly predicting protein structure. Such advances, although in apparently distant and disparate disciplines, are relevant. Arbitration is, ultimately, a type of game, playable just as Go is; arbitration games must therefore be, theoretically, machine-solvable. Theoretical modelling of alternative dispute resolution by game theorists, including of arbitration as a Bayesian game, has led, for example, to proposals that there can be an optimal protocol for arbitration, which is deterministic.8)Goltsman, M., J. Horner, G. Pavlov and F. Squintani (2009). “Mediation, arbitration and negotiation.” J. Econ. Theory 144: 1397-1420. And arbitration is a form of complex problem. If a problem previously thought unsolvable because of its complexity has been cracked by AI (such as protein folding), designing an artificially intelligent arbitral participant may not be impossible. Slightly reassuringly for pessimists, it has been shown that the popular card game Magic: The Gathering is at least as difficult as Turing’s halting problem, and that optimal strategy is non-computable, nor is evaluating consequences of prior moves.
Risk of harm
Not all AI helps arbitration; certain flaws may harm it. As with the expression “rubbish in, rubbish out”, the quality of the AI output relies on the quality of the input. A poor training set may hinder technology-assisted review and, over a large dataset, may promote increasing inefficiency. Deliberate action, such as data poisoning, the injection of false training data with the aim of corrupting the learned model, is also a real threat for any AI system.9)Steinhardt, J., P. W. Koh and P. Liang (2017). Certified defenses for data poisoning attacks. Proceedings of the 31st International Conference on Neural Information Processing Systems. Or, bias can be hard-coded into datasets. For example, it has long been known that men and women use language differently.10)Newman, M., C. J. Groom, L. D. Handelman and J. Pennebaker (2008). “Gender Differences in Language Use: An Analysis of 14,000 Text Samples.” Discourse Processes 45: 211 – 236. Any user of such technology must recognise and allow for its shortcomings.
Another difficulty lies in the algorithmic basis of AI. Unlike a reasoned human being, how or why a result was achieved is not always known (or knowable). In the only reported case in England to date, an automated decision-making process for investments was in question, whereby the extent of control and knowledge over the AI system was apparently unknown. See Tyndaris v MMWWVWM [2020] EWHC 778 (Comm). Challenging questions remain of due process in automation and transparency, particularly if the reasoning applied might not be divined – unfortunately, Tyndaris was struck out for procedural reasons.
A further difficulty lies in evidence. As AI systems improve, evidence will become easier to falsify, and fakes harder to detect. The rapid development in synthetic media such as “deepfakes” has led to rapid research and development in this area.
Respice In Posterum
As the late Professor Gaillard wrote, at the heart of it, arbitration is the will and liberty of the parties. In this sense, AI can neither help nor harm it. If the parties freely choose to involve or restrict AI, in any aspect of their arbitration, that is a choice validly made and to be respected. Indeed, it may be that restricting the use of AI could pose its own challenges. For example, would that deprive a party who is unable to proceed without AI assistance of a fair trial? In an extreme case such as this, it might not matter that the reasons for the ultimate decision could be unknown. Or, as Lord Neuberger once said, quick and dirty justice is better than the risk of no justice at all.11)The Evening Standard, 15 November 2013 (Evening Standard).
=======
Further posts on our Southeast and East Asia’s Think Arbi series can be found here. This limited series showcases short versions of selected thought leadership pieces from our next-generation arbitration practitioners.
________________________
To make sure you do not miss out on regular updates from the Kluwer Arbitration Blog, please subscribe here. To submit a proposal for a blog post, please consult our Editorial Guidelines.
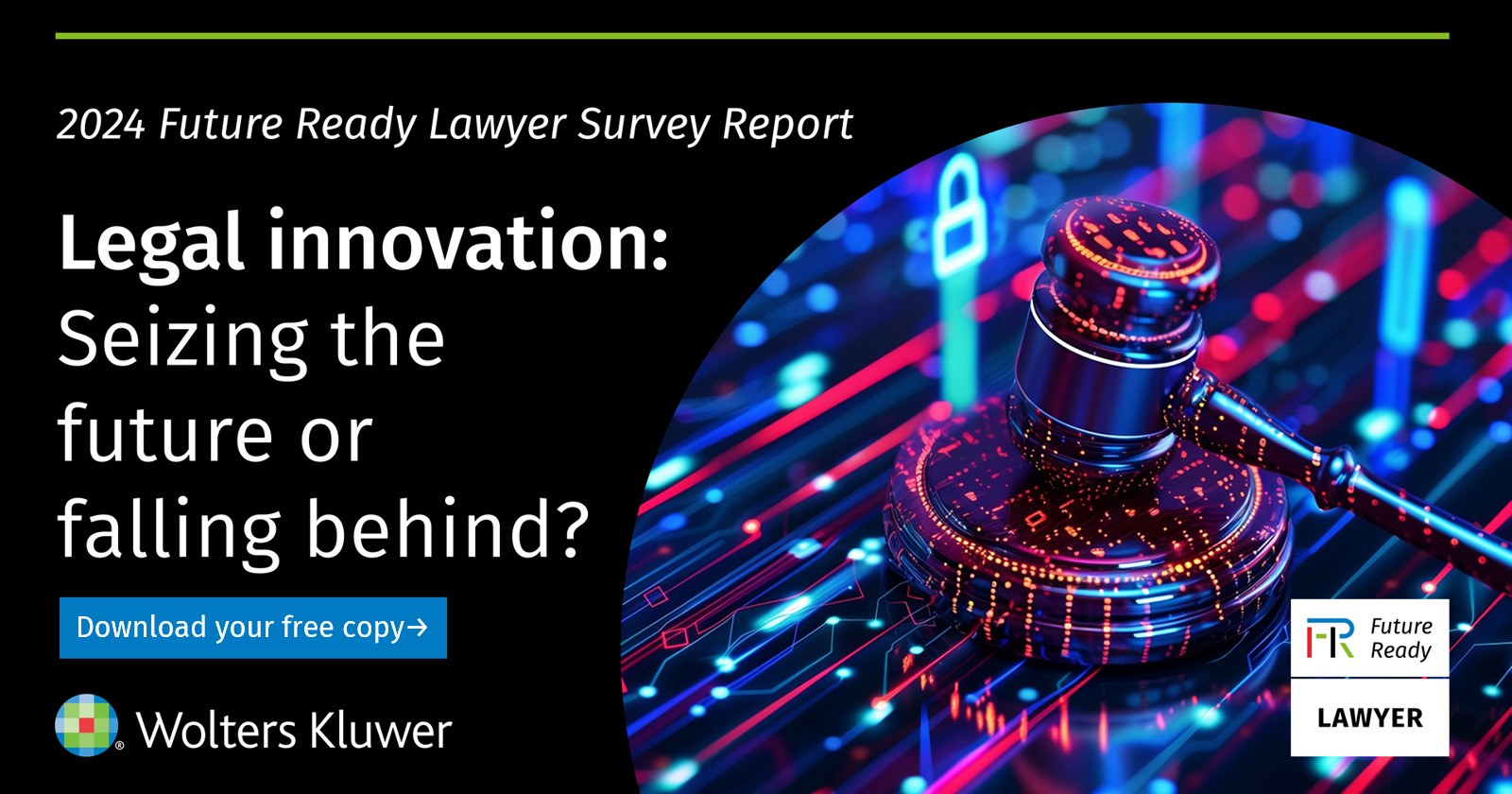
References
↑1 | Kerr, L. J. (1987). “Arbitration v. Litigation: The Macao Sardine Case.” Arbitration International 3(1): 79-86. |
---|---|
↑2 | Turing, A. (1937). “On Computable Numbers, with an Application to the Entscheidungsproblem.” Proceedings of The London Mathematical Society 41: 230-265. |
↑3 | McCarthy, J., M. Minsky, N. Rochester and C. Shannon (1955, August 31). “A Proposal for the Dartmouth Summer Research Project on Artificial Intelligence.“ |
↑4 | Newell, A. (1973). “You can’t play 20 questions with nature and win: projective comments on the papers of this symposium.” Visual Information Processing: 283–308. |
↑5 | See also Brown v BCA Trading Ltd [2016] EWHC 1464 (Ch), where the order was contested. In other jurisdictions: in Australia, McConnell Dowell Constructors (Aust) Pty Ltd v. Santam Ltd (No 1) [2016] VSC 734; Vickery J. at [18] to [31] (1.4 million documents); in the US, Da Silva Moore v Publicis Groupe 11 Civ.1279 (ALC)(AJP), 24 February 2012, Southern District of New York (3 million documents); in Ireland, Irish Bank Resolution Corporation Ltd v Quinn [2015] IEHC 175; Fullam J. at [17] to [30] (1.7 million documents). |
↑6 | Katz, D. M., M. J. Bommarito and J. Blackman (2017). “A general approach for predicting the behavior of the Supreme Court of the United States.” PLoS One 12(4). |
↑7 | Shaikh, R. A., T. P. Sahu and V. Anand (2020). “Predicting Outcomes of Legal Cases based on Legal Factors using Classifiers.” Procedia Computer Science 167: 2393-2402. |
↑8 | Goltsman, M., J. Horner, G. Pavlov and F. Squintani (2009). “Mediation, arbitration and negotiation.” J. Econ. Theory 144: 1397-1420. |
↑9 | Steinhardt, J., P. W. Koh and P. Liang (2017). Certified defenses for data poisoning attacks. Proceedings of the 31st International Conference on Neural Information Processing Systems. |
↑10 | Newman, M., C. J. Groom, L. D. Handelman and J. Pennebaker (2008). “Gender Differences in Language Use: An Analysis of 14,000 Text Samples.” Discourse Processes 45: 211 – 236. |
↑11 | The Evening Standard, 15 November 2013 (Evening Standard). |
