In modern arbitration proceedings, most evidence goes through a digital process before reaching the eyes of decision-makers. Physical documents are scanned, processed with Optical Character Recognition (“OCR”), archived, and transferred. Digitally created documents follow the last two steps as well. Sometimes these processes are repeated multiple times. This “assembly line of evidence” has become the norm, with little questioning.
So far, concerns surrounding digitally created or recorded evidence focus on human factors, such as the creation of deepfakes or the hacking of evidence. But what happens when the technology itself manipulates evidence without any direct human intervention?
This blog post considers the risks of digital evidence manipulation, such as image and video enhancements, errors in OCR, compression algorithms, and automatic tagging (“auto-tagging”), which can distort or alter evidence. It emphasises the need for careful assessment of digital evidence to ensure its accuracy and reliability in arbitration proceedings.
Enhanced Videos and Images
A notable example of the challenges surrounding enhanced videos and images is the debate in Wisconsin v Kyle Rittenhouse, Kenosha County, State of Wisconsin. In this case, the prosecution submitted as evidence a drone video recording depicting the moment when the defendant shot one of the victims. As the timing of events was particularly important to proving self-defence, the prosecution requested to zoom in on the drone footage for better quality, using an iPad (an Apple product). The defence objected, arguing that when zooming in, Apple’s algorithm enhances images by adding pixels, effectively “creating what it thinks is there, not what necessarily is there.” As a result, the defence claimed that the evidence no longer represented a true depiction of facts.
This objection sparked doubt for Judge Schroeder, who expressed concerns about the accuracy of the zoomed-in image. He requested that the prosecution provide an expert to validate the accuracy of the enhanced footage. However, the prosecution was unable to do so within the, albeit very short, allocated time of 15 minutes.
The court ultimately held that the burden of proof lay with the prosecution, as the proponent of the exhibit, to show that the zoomed-in image did not distort the object depicted. As the prosecution could not bring an expert to testify that the zoom-in did not alter the video’s accuracy, the evidence was presented in its original size and format on a Windows device. Before reaching a verdict, the jury asked to rewatch all the video evidence submitted for the case (including the drone footage), and eventually acquitted the defendant on all charges.
The case sparked a lot of debate online. Some commentators criticised the judge for failing to understand how technology works and for the limited time given to the prosecution to find an expert witness. Others agreed with the defence, pointing out that pinch-to-zoom uses algorithms to add pixels and enhance videos through interpolation. Such enhancements can introduce distortions, which may be critical in situations where figuring out the precise moment when something happened is of the essence. In those situations, every pixel added could make a difference.
While the case concerned criminal proceedings, similar issues can arise in arbitration, where video evidence is decisive in resolving disputes. Just like in criminal cases, arbitral tribunals may face challenges regarding the authenticity and accuracy of enhanced video and photo evidence.
Similar issues are likely to appear more often in the future considering most modern cameras and smartphones come with built-in features that automatically enhance photos and videos. These features, often enabled by default, adjust brightness, contrast, and colour balance without user input, potentially altering the original appearance of the footage. For example, according to Samsung, “When you take a photo of the moon with your Galaxy device, the camera system uses deep learning-based AI, along with multi-frame processing, to enhance details.”
Inaccurate OCR
OCR is a commonly used tool that converts scanned documents into readable and searchable digital text. However, OCR software can misinterpret characters, especially when working with poor-quality scans, unusual fonts, or less commonly used languages. This can lead to inaccuracies in the digital text. Additionally, annotations and handwritten notes might be lost in the process. For example, Adobe Inc. is not shy in admitting that “OCR is an imperfect process,” as well as that it “affects search quality and that should be a concern to legal professionals.”
Despite its usefulness, OCR can create real issues in dispute resolution. For example, in 2015, the High Court of England and Wales in Smailes v McNally [2015] EWHC 1755 (Ch) sanctioned one party for failing to meet its disclosure duties due to relying on defective OCR. More specifically, during the disclosure stage, the claimant relied on scanned versions of physical documents that had been processed through OCR and uploaded to a dedicated platform. However, the OCR results were of low quality and riddled with inaccuracies. This led to an incomplete set of documents being identified as relevant when keyword searches were applied. The court deemed this a serious and significant failure to carry out a reasonable search, therefore emphasising the importance of ensuring the accuracy of OCR-generated text in legal proceedings.
Loss of Data Integrity with Compression Algorithms
The cases of Wisconsin v Kyle Rittenhouse and Smailes v McNally are not unique examples where concerns surfaced about technology distorting digital information. In 2013, computer scientist David Kriesel found that Xerox scanners changed numbers on documents, sometimes even adding new information that differed from the original physical documents. Kriesel concluded that the issue was not caused by OCR but rather by the scanner’s embedded image compression standard, JBIG2. JBIG2 uses lossless and lossy compression. Lossy compression reduces file size by permanently removing data. JBIG2’s lossy compression can alter characters in scanned documents by using “pattern matching” to identify similar symbols. Poor matching, especially in low-resolution scans, can lead to incorrect character swaps and data loss. Xerox acknowledged that “[t]he problem stems from a combination of compression level and resolution setting.” Kriesel’s findings prompted the German Federal Office for Safety in Information Technology to ban the use of JBIG2 for archival purposes.
The risks of data loss are not confined to a single type of compression algorithm. The UK National Police Chiefs’ Council and the Defence Science and Technology Laboratory mention in their Guidance Digital Imaging and Multimedia Procedure v3.0 that “[a]ll compression algorithms remove data.” They can inadvertently alter the quality of the original file, introducing fake details, known as “artifacts,” that distort the image or reduce the clarity of its content.
Unfortunately, attempts to retrieve or reconstruct data chains are not always efficient, and can adversely impact the quality of the information depicted, potentially compromising its reliability in critical contexts.
Erroneous Auto-Tagging
Automatic tagging is another useful, but also potentially risky, feature. It employs object recognition and categorisation based on various criteria to organise information when dealing with numerous pieces of documentation. However, when used without human oversight, it can lead to problematic results. For example, in 2015, Google faced widespread online criticism when its Google Photos app mistakenly labelled people of colour as gorillas.
In arbitration, auto-tagging can help manage large volumes of evidence, but without proper oversight, it can lead to the misclassification or exacerbation of biases. For instance, object recognition algorithms might mislabel or misclassify key evidence, leading to inaccurate or incomplete document disclosure. This can affect the accuracy of searches, the identification of key evidence, and ultimately, the fairness of the arbitration process.
Call to Action for Qualitative Decision-Making
There is a tendency to overly rely on automated systems for evidence collection, presentation, and analysis. This dependency can lead to complacency in verifying the integrity of the evidence. Many stakeholders may not fully appreciate how automated systems can compromise the integrity of evidence, such as emails, videos, and scanned documents without direct human intervention or malicious intent.
While digital evidence is ubiquitous in international arbitration, we often assume it is identical to physical (or “real”) evidence. The examples above show that this is not always the case. Questions regarding the authenticity, admissibility, reliability, burden of proof, and potential loss of “veracity” of digital evidence are ever more pressing.
Qualitative decision-making requires both a familiarity with the technology involved and a solid understanding of the challenges associated with digital evidence. Still, there is little to no guidance on how to handle digital evidence, or how to avoid and mitigate the types of technological interference analysed in this blog post.
International organisations and arbitral institutions could play a key role in setting best practices for digital evidence in arbitration. Standardised guidelines could cover issues such as the assessment, validation, and presentation of evidence, as well as protocols for gathering and transferring data, and recommendations addressing technological interference and data loss. Such guidelines would provide tribunals with a clear framework to ensure the authenticity and reliability of digital evidence, enhancing the fairness and integrity of arbitration proceedings.
________________________
To make sure you do not miss out on regular updates from the Kluwer Arbitration Blog, please subscribe here. To submit a proposal for a blog post, please consult our Editorial Guidelines.
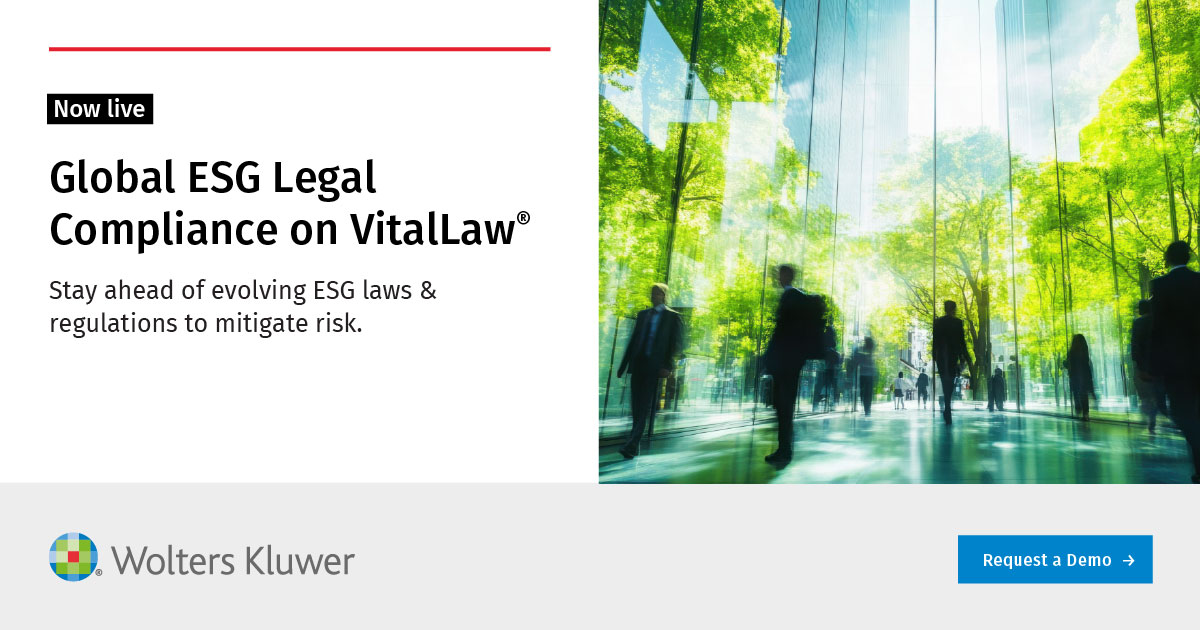
