A black box artificial intelligence (“AI”) model is one “created directly from data by an algorithm, meaning that humans, even those who design them, cannot understand how variables are being combined to make predictions” (see a more detailed discussion here). That we do not understand the way an AI reaches its conclusions is creating discomfort over the implementation and use of AI. We first summarise the views shared in two recent seminars by Young ICCA and Maxwell Chambers. We then turn to share our thoughts on the same.
Young ICCA
On 29 March 2023, within the framework of the Young ICCA mentoring program me and sponsored by ArbTech, the webinar Will I Lose My Job to a Robot? took place. The event sparked a robust discussion concerning the hype around new cutting-edge tools based on AI and their applications in international arbitration.
Sofía Klot (Senior Associate, Freshfields Bruckhaus Deringer) discussed which predictive and generative AI tools are increasingly being used in arbitration and litigation (e.g., ArbiLex; Casetext – CoCounsel; Harvey and productivity tools such as Microsoft’s Co-Pilot). She also raised two key issues: (1) the use cases for AI in arbitration and (2) ethical and legal risks.
- Large Language Models (“LLMs”) consist of neural networks trained on large amounts of text that are very good at anticipating, generating and predicting language. They can be used to create tailor-made outputs for a range of applications, including for arbitration. Klot pointed to several ways in which lawyers can use LLMs, along with new career paths (e.g., in data annotation and labelling), and explained how arbitrators and arbitral institutions can also leverage LLMs (e.g., to summarize parties’ positions, automate transcriptions of hearings, and prepare the procedural history of a case for publication in an award); and
- Ethical and legal risks posed by AI (for instance, preserving client data confidentiality and preventing the use of fake evidence). (Such issues are explored in further detail on the Blog here.)
The second discussion was led by Emily Hay (Counsel, Hanotiau & van den Berg) and Federico Ast (Founder & CEO, Kleros). After a thorough overview of blockchain and Kleros, the discussion turned to how domestic courts will react to blockchain-based awards in AI-related disputes.
Considering the New York Convention, Hay outlined challenges in: (i) identifying an arbitral seat; (ii) lack of reasoning in self-enforceable awards; (iii) complying with the writing requirement of the arbitration agreement; (iv) having an original and duly authenticated award; and (v) non-compliance with due process (discussed further on the Blog here). Ast, in turn, brought to the table a Kleros case that overcame those barriers: in 2021, a Mexican court enforced a blockchain-based award by incorporating it by reference into a traditional arbitration award (discussed on the Blog here).
Finally, Mauricio Sánchez Lemus (Contract International Advisor, White & Case LLP) addressed the AI scheme from a regulatory perspective. He analyzed the reaction of states to AI-based technologies and the problems they entail. For Sánchez Lemus, states are running this race far behind the speed at which AI tools are growing.
Maxwell Chambers
On 27 April 2023, as part of its inaugural #BeyondtheBlackBox series, Maxwell Chambers hosted a closed-door roundtable discussion under Chatham House Rule on the implementation and use of AI. Participants were treated to a live demonstration of Rocketeer – an AI tool that can predict the outcome of a conflict between trademarks.
A topic discussed at length was how the use and implementation of AI could potentially stifle legal innovation and accelerate tunnel vision. AI largely relies on the use of robust datasets, patterns, and predictive learning. However, this very nature of AI seems antithetical to the conditions required for legal innovation. Seminal cases like Donoghue v Stevenson suggest that critical analysis and courage to depart from established principles are necessary for legal developments.
Another point raised in the discussion is that AI does not need to reach perfection before it is preferred over human input in a wide variety of contexts. For example, if AI can predict with just 80% accuracy the likely outcome of a dispute based on inputs of fact and evidence, businesses may consider that preferable to costly legal advice—the traditional antecedent to the commencement of legal proceedings.
Nevertheless, the majority agreed that AI holds great potential and possibilities for the legal sector. Possible uses of AI tools in international arbitration and dispute resolution, such as ChatGPT, are being explored (as discussed here, here and here).
Our thoughts
Inherent nature of AI and “AI Singularity”: will this mean more arbitrations over misplaced regulations?
With the rapid speed at which AI is developing, governments are scrambling to put in place regulations to curb and control the use of AI. Chinese regulators recently released draft rules designed to manage how companies develop generative AI products like ChatGPT, with the aim of circumscribing AI development. For example, under these rules, the content generated by AI needs to reflect the core values of socialism and should not subvert state power. Similarly, the UK government recently published a white paper on the future of governance and regulation of AI.
The rush to impose regulations stems from an assumption that the concerns brought about by AI can be resolved with a better understanding of how AI works and effective control of undesirable outcomes.
However, the inherent nature of AI makes this difficult. Current AI systems use artificial neural networks that mimic the complex organisational structure of deep neural networks in the human brain. The human brain is already difficult to understand. It is unsurprising then, that even the people behind the development of AI are facing problems explaining how it works and why certain outputs are generated. We may have to accept that we cannot control or manage something we do not understand.
The greatest discomfort with AI is its potential to eventually evolve into something we can no longer control or restrain, simply because we cannot grasp how it works. Indeed, the concept of “AI Singularity” was raised in the roundtable discussion. AI Singularity refers to the tipping point at which AI systems become so advanced that they transcend human intelligence. At this point, humanity becomes unable to understand or control the very technology it creates, potentially resulting in a future where humans and human innovation become obsolete. Experts do not know when this can happen, but the possibility is certainly concerning.
Regulators thus must take this into account when implementing legislation. No doubt arbitration practitioners will watch this space carefully for disputes arising out of misplaced regulations. One possible (but extreme and potentially controversial) way to tackle this is for regulators to move fast and strictly curtail the ability for private parties to develop AI. This may arguably be what Chinese regulators are now doing. However, we need to decide how far we should let our fear of the unknown limit the potentially revolutionary developments we could make as a human race.
Can predictive AI tools enhance the decision-making process?
AI poses several challenges that should keep us on our toes. LLMs lack awareness and creative capabilities are limited to the data sets they are fed (“garbage in, garbage out”). The absence of biases is crucial when deciding to incorporate them. Therefore, parties and arbitrators can be concerned about simply “outsourcing” the decision-making function to predictive algorithms. When using predictive AI, it is vital to detail its usage in the arbitration agreement, and ask questions such as: how is the training data selected and labelled? Are there auditing procedures built into the system? How is the data updated? Are there controls for algorithmic biases?
Furthermore, AI systems with invisible inputs and inner workings might generate conclusions without providing explanations as to how they were reached (the black box phenomenon). The solution may lie in incorporating explainable AI into the algorithm. However, machine learning algorithms sometimes do not base their “decisions” and predictions on the applicable law or facts of the case, but on information that humans would not find relevant.
Moreover, AI poses practical challenges in arbitration:
- confidentiality and data management: client data could need to be stored in a private server, cloud or data room; it should be anonymized and should not be fed back into the training algorithm;
- manipulation of evidence; and
- infringements of the equality of arms principle.
While AI will not replace certain human cognitive functions, it will increasingly permeate our practice. We must be prepared to be at the forefront and respond to clients’ needs.
Conclusion
The implementation and use of AI carries both risks and rewards, and not just in the legal sector. One way in which governments are attempting to manage the risks is through regulation and careful supervision. But with growing interest and rapid developments in AI, coupled with the inherent limits to human understanding of complex neural AI systems, it remains to be seen whether we can continue being the masters of the very thing we created.
Further posts in our Arbitration Tech Toolbox series can be found here.
The content of this post is intended for educational and general information. It is not intended for any promotional purposes. Kluwer Arbitration Blog, the Editorial Board, and this post’s author make no representation or warranty of any kind, express or implied, regarding the accuracy or completeness of any information in this post.
________________________
To make sure you do not miss out on regular updates from the Kluwer Arbitration Blog, please subscribe here. To submit a proposal for a blog post, please consult our Editorial Guidelines.
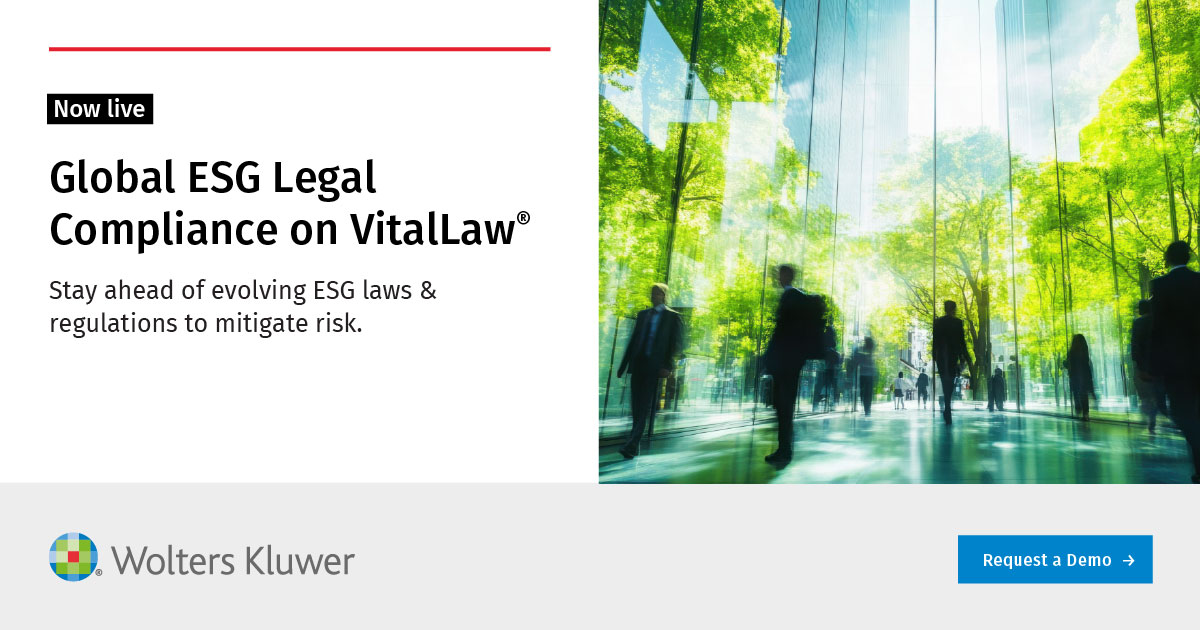
