Artificial Intelligence (“AI”) is the buzzword of the day. It has crept into every pore of society, and arbitration has not evaded this trend. The main question raised by commentators and the public is whether AI will render human involvement obsolete, or at least reduce it to a bare minimum. In the context of arbitration, this question translates to: Will AI be able to act as a tribunal secretary or – even more so – as an arbitrator, thus substituting human arbitrators? Two prominent AI studies that were relatively successful in predicting outcomes of court judgments of the European Court of Human Rights (“ECtHR”) and the Supreme Court of the United States (“SCOTUS”) and took place in 2016 and 2017 respectively, sparked curiosity over this matter.
The potential use of AI as an arbitrator in real disputes has been linked with several risks. For instance, the effectiveness and reliability of an AI system depend on the availability of vast data sets for training. In arbitration, it is questionable whether such large data sets would be available because most arbitral awards remain confidential. In investment arbitration, this problem is even more pronounced since the overall number of awards rendered per year is low.
One potential solution in international commercial arbitration is training AI arbitrators on court judgments, as parties often select specific national laws to govern their transactions. However, it is questionable whether this would be a viable solution for several reasons. Not all jurisdictions make court judgments, especially from lower courts, readily available. Additionally, national laws and court judgments would be of little use in training an AI arbitrator when it comes to procedural issues, such as evidentiary issues, as different rules apply in arbitration. One may add to these concerns the risk of judicialization of arbitral proceedings. This term is mostly used to refer to the increasing resemblance of arbitral procedures to court proceedings, but it can also be used in relation to substantive issues. Arbitrators might be more flexible and liberal in their approach to these issues, especially if acting ex aequo et bono. Training AI arbitrators strictly on court judgments may eliminate this flexibility, leading to less effective arbitration.
Another significant risk is bias. The data used to train an AI system may be tainted with historical and human biases, which could be reflected in AI-generated decisions. Some have emphasized the importance of empathy and human interaction in dispute resolution, something that AI is not capable of, even if it can be programmed to replicate human emotional responses. Moreover, the “black box” problem presents a major obstacle to having AI serve as an arbitrator because of its supposed incompatibility with the current legal framework for international arbitration and due to the perception that the lack of transparency can undermine trust in AI-generated decisions.
While all these risks are worthy of further exploration, this blog post will address one particular risk – that of the “black box” problem.
What Is the “Black Box” Problem?
In the AI domain, the “black box” problem means that the path the AI model takes to reach a result is not identifiable. This means that, while AI can produce results, the specific methods and factors it uses to get these results are not fully understood. This is particularly true for machine learning models, which autonomously find patterns in large volumes of data and reach an outcome.
The “black box” problem poses a significant challenge to having AI serve as an arbitrator. Even if an AI model could be designed to render decisions in arbitration cases, the exact factors and patterns it considered to reach its conclusions would remain unknown. A potential solution to this conundrum has been devised in the form of the so-called “Explainable AI” which not only provides desired outputs, but also explains how it generated those outputs. However, Explainable AI comes with significant trade-offs, and the more explainable the AI, the more its precision and reliability may be sacrificed in the name of transparency.
The “Black Box” Problem and the Current Legal Framework in Arbitration
The “black box” problem stands as a hurdle for the use of AI as an arbitrator primarily because the current international arbitration legal framework requires reasoned awards. Arbitrators are generally expected to put forth reasons that explain why they reached a particular outcome in the award.
To illustrate, Article 31(2) of UNCITRAL Model Law on International Commercial Arbitration provides that “[t]he award shall state the reasons upon which it is based, unless the parties have agreed that no reasons are to be given or the award is an award on agreed terms […].” The UNCITRAL Arbitration Rules align with the Model Law, providing in Article 34(3) that “[t]he arbitral tribunal shall state the reasons upon which the award is based, unless the parties have agreed that no reasons are to be given.”
Similar approaches are found in laws that are not based on the UNCITRAL instruments, as well as in the rules of prominent arbitral institutions. For instance, Section 52(4) of the English Arbitration Act 1996 states that “[t]he award shall contain the reasons for the award unless it is an agreed award or the parties have agreed to dispense with reasons.” Article 32(2) of the ICC Rules also requires that “[t]he award shall state the reasons upon which it is based.”
Imagine the Following Scenario
Is the “black box” problem an insurmountable obstacle for AI arbitrators? The answer may very well depend on whether the arbitration community would accept AI-generated decisions without fully knowing or understanding how they were reached. Let us assume that AI has attained a level of development whereby it can determine with high accuracy the outcomes of arbitration cases. We can also consider the easy versus hard cases dichotomy as espoused by H.L.A. Hart. Easy cases have clear rules the application of which leaves very little, if any, manoeuvring room for differing interpretations and outcomes. Hard cases involve several defensible positions that may be espoused, each equally plausible.
Imagine a spectrum with easy cases on one end, hard cases on the other, and intermediate cases that could gravitate towards either one or the other side. Now, assume that we have an AI model that renders the correct outcome in 100% of the easy (or easiest) cases. As cases become more complex and gravitate towards the hard case categorization, the AI starts reflecting differing opinions within case law. However, let us assume that for hard cases, the AI arbitrator would align with the majority view. In other words, if in the hardest of cases, out of 11 human arbitrators, six would decide the case one way while the remaining five would take the opposite approach, the AI arbitrator would decide as the former.
The fundamental question is whether parties would be willing to engage in this kind of decision-making process and accept the outcome as final and binding. After all, an AI arbitrator could offer some palpable advantages over human-led arbitration, such as reduced costs and faster resolution times. An AI arbitrator would presumably be in a position to render an outcome fairly quickly (AI processes data much faster than humans), and for only a fraction of the cost compared to human arbitrators. Given these purported advantages, it would be informative to conduct empirical research amongst arbitration stakeholders to gauge their willingness to opt for AI arbitrators in spite of the “black box” problem, based on the assumptions outlined above. It is plausible that parties could be open to resorting to AI arbitrators for lower value and less complex cases, which, on the spectrum of easy v. hard cases, gravitate towards the former.
Change of Paradigm?
Looking at the current legal framework for international arbitration, would a change of paradigm be needed to accommodate AI arbitrators in spite of the “black box” problem? After all, fully reasoned arbitral awards play a significant role in international arbitration, by helping parties understand and accept why a particular decision has been reached. They serve as a check on arbitrariness, ensuring that awards are logical and based on sound reasons. Moreover, reasons may provide grounds for the losing party to successfully have the award set aside or to resist its recognition and enforcement.
However, reasoned awards are not an absolute requirement in the world of arbitration. Both the UNCITRAL Model Law and the Arbitration Rules require that the awards contain reasons, but only as far as the parties have not agreed otherwise. This suggests that the parties could agree to accept the decision of an AI arbitrator without traditional reasoning or with reasoning that does not reveal the exact patterns in data that had been relied upon by the AI system.
Of course, the fact that reasoned awards are not an absolute requirement within the arbitration framework does not in any way diminish the importance of what they aim to ensure. Thus, to foster broader acceptance of AI arbitrators, it would be advisable to take steps to address the concerns traditionally addressed by reasoned arbitral awards. These issues could be tackled before the award is rendered. For instance, the institution behind the AI arbitrator could maintain the transparency of the data set used to train the AI or the measures taken to tackle biases in the data set.
While these ex ante solutions may not be foolproof, one must remember that neither are reasoned awards. Legal skeptics have long been pointing out that decision-makers such as arbitrators or judges may well decide an outcome first (that may or may not be impacted by their biases), and afterward formulate justifications, which may not necessarily reflect the true reasons why they decided the case in one way over another. Ironically, AI arbitrators may be a lesser “black box” than human minds.
Further posts in our Arbitration Tech Toolbox series can be found here.
________________________
To make sure you do not miss out on regular updates from the Kluwer Arbitration Blog, please subscribe here. To submit a proposal for a blog post, please consult our Editorial Guidelines.
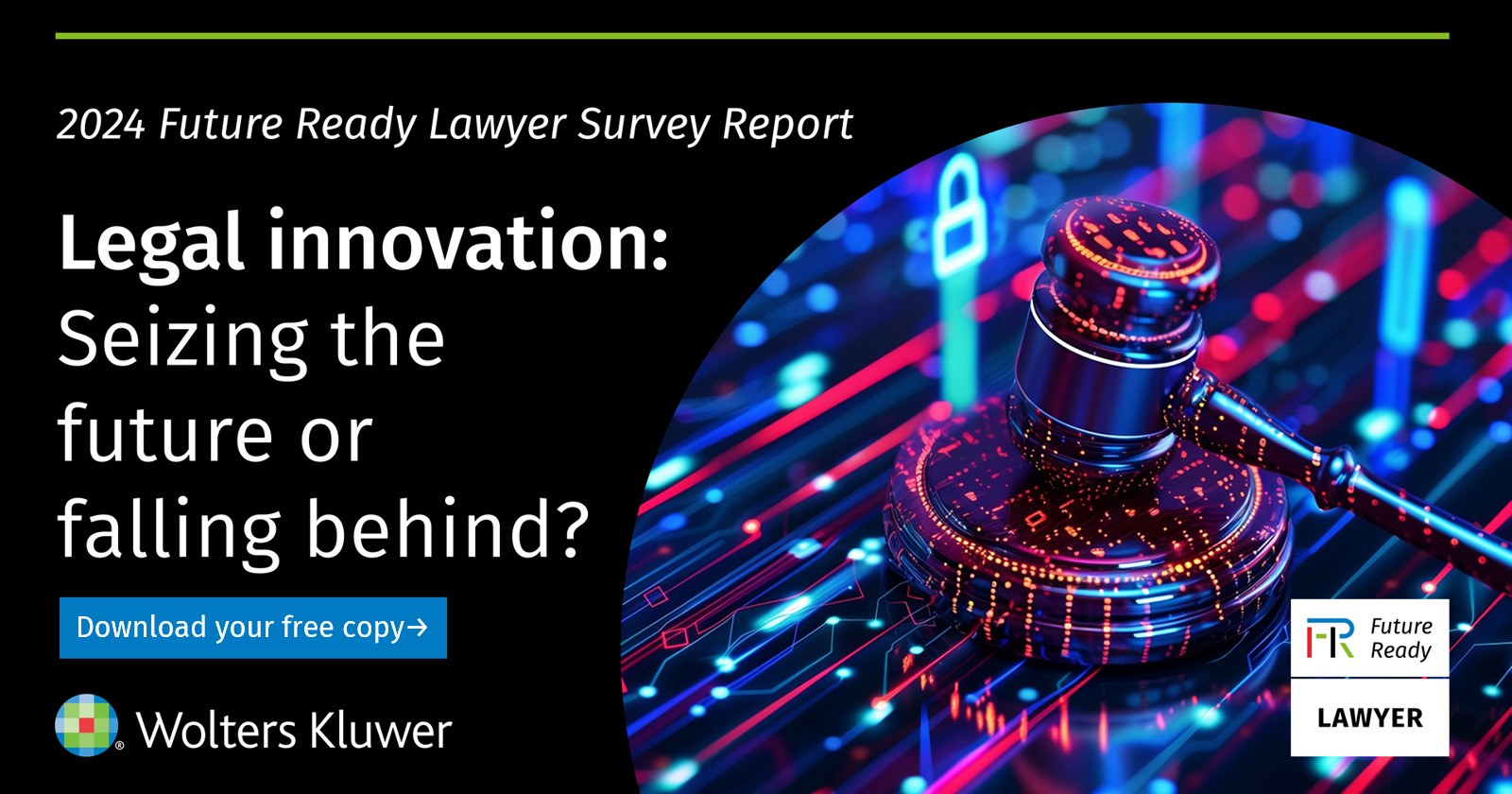
